We are SNGULAR
We're not the average tech partner. We're the next-generation innovators, proving that the unconventional delivers.
With decades of expertise in bringing groundbreaking technology services and projects to life for industry leaders, we're not content with the ordinary. We dare to disrupt and transform visionary ideas into reality.
Our secret? We combine deep technical expertise with talent and tenacity to always deliver.
A general vision in numbers
-
+1300
People
Since December 2023.
-
+500
Clients
In 11 regions.
-
+900
Projects
Annually.
-
+100
million
In revenue in 2023.
Our latest news
Interested in learning more about how we are constantly adapting to the new digital frontier?
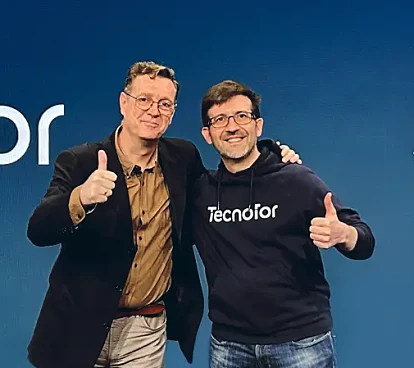
Corporate news
April 25, 2024
SNGULAR acquires TecnoFor, Atlassian Platinum Partner and leader in corporate technology training
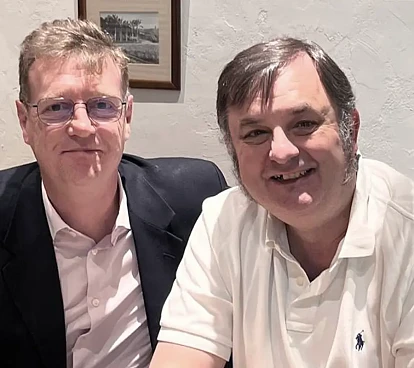
Corporate news
April 22, 2024
Javier Recuenco, new Strategy Advisor at SNGULAR
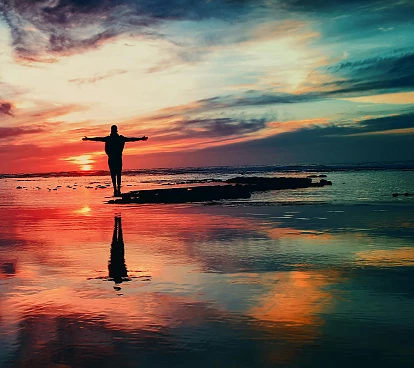
Insight
April 16, 2024
Leave a mark on people, not on the planet
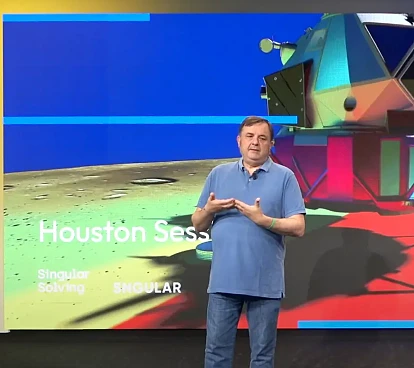
Corporate news
April 11, 2024
Why Unconventional CPS Had to Be Created
Contact us
Would you want our SNGULAR people to contact you?
Leave us your details and we will get in touch.